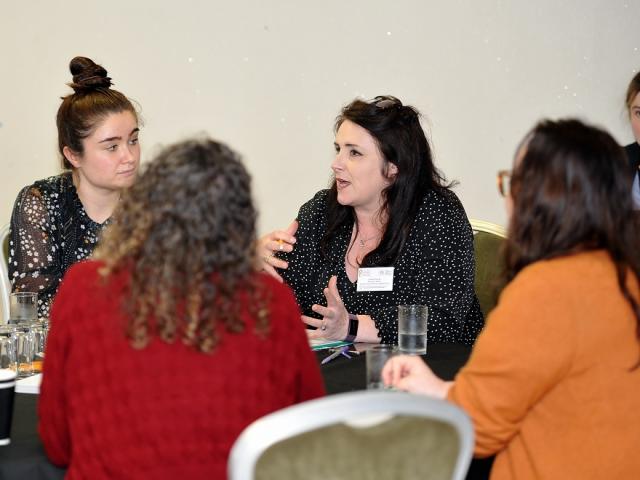
SAIL Databank Showcase Webinar Series April 2024
Mae’r cynnwys hwn wedi dod o wefan allanol ac mae ar gael yn Saesneg yn unig.
The Secure Anonymised Information Linkage (SAIL) Databank Showcase Webinar Series is a great opportunity to hear from guest speakers about the research they're undertaking and to get all the latest information and ask questions across the SAIL Databank team and group leads, including infrastructure, analytical services, data resources and documentation, public engagement, business and other developments and updates.
The day's agenda is as follows:
10:00-10:10
Ashley Akbari & Chris Orton – Population Data Science, Swansea University
Welcome and Introductions.
10:10-10:30
Miss Jane Lyons, Administrative Data Research Wales, Population Data Science, Swansea University
Using multi-state models to measure trajectories in chronic disease accrual and mortality across the lifespan in Wales, UK, to identify disparities in health outcomes in our society.
Understanding and quantifying the differences in disease development in different socioeconomic groups of people across the lifespan is important for planning healthcare and preventive services.
Our population-wide electronic linked cohort study aimed to measure chronic disease accrual, and examine the differences in time to individual morbidities, multimorbidity, and mortality between socioeconomic groups in Wales, UK.
Multi-state models were used to examine trajectories of accrual of 132 diseases and mortality over 15 years (2005-2019) for the population of Wales, adjusted for sex, age and area-level deprivation. Restricted mean survival time was calculated to measure time spent free of chronic disease(s) or mortality between socioeconomic groups.
10:30-10:50
Prof Simon Fraser and Prof Nisreen Alwan, University of Southampton
MELD-B – a lifecourse approach to multimorbidity prevention using multiple data sources
MELD-B is one of seven NIHR AIM Research Consortia using AI and other methods to study various aspects of multimorbidity. We will present on how we are using AI and causal inference methods to investigate the development of multiple long-term conditions across the lifecourse, with a particular focus on early life determinants. We will describe our use of two large electronic health record sources (SAIL, CPRD) and three birth cohorts.
We have a particular interest in multimorbidity that is ‘burdensome’ for people and will describe this further in our presentation.
10:50-11:10
Professor Rhiannon Owen, Swansea University
Modelling trajectories of disease in multiple long-term conditions using electronic health records.
People are increasingly living with multiple long-term conditions, also known as multimorbidity. Multimorbidity can encompass many different combinations of long-term conditions, and these combinations can cluster in a concordant or discordant manner. The epidemiology of multimorbidity has to date concentrated on the static clustering of diseases with respect to time rather than their dynamic evolution or trajectory over the life course. This talk will discuss the use of multistate models to examine how common clusters of disease develop over time. The models are applied to the Wales Multi-morbidity e-Cohort (WMC) in the SAIL Databank. The WMC includes population scale, individual-level, anonymised, linked, multi-sourced data, including demographic, administrative and electronic health records for 2.9 million individuals. Using a case study in psychosis, diabetes, and congestive heart failure, this presentation will discuss the impact of different trajectories of disease on mortality, identify potential screening and therapeutic targets, and assess potential risk factors in physical-mental health multimorbidity.
11:10-11:30
Dr Emeka Abakasanga, Loughborough University
Identification of Multiple Long-term Condition Clusters and Equitable Length of Hospital Stay Prediction for Adults with Learning Disabilities
There are approximately 1.1 million UK adults aged 18+ living with a learning disability (LD). Individuals with LDs often experience poorer physical and mental health, as well as higher comorbidity rates and avoidable mortality than those without LDs. This talk presents recent findings from the NIHR-funded project entitled DECODE: using machine learning to Identify Clusters of multiple long-term conditions (MLTCs) in Individuals with LDs. This talk will cover:
1) Development of a machine learning model with effective bias mitigation approaches to predict hospital length of stay by addressing data imbalances across ethnic groups.
2) Identifying clusters of MLTCs using machine learning and statistical approaches.
11:30-12:00
Updates from SAIL Databank teams.
This conference is being run by The SAIL Databank if you have any questions, please contact the event team.
Free